Linear programming (LP) is, as already said in a previous post about linear programming, a mathematical solution.
Basically, in linear programming, we turn our practical issue into a logical model. It includes a subjective restriction, linear inequality, and an objective function.
In LP, there are constraints that can be positive or negative. Wondering what happens if they are negative? Well, can they even be negative?
In this article, you will find out more about linear programming! To be exact, find out if linear programming can be negative, and what can you do with such programming.
Advertising links are marked with *. We receive a small commission on sales, nothing changes for you.
Elements of Linear Programming
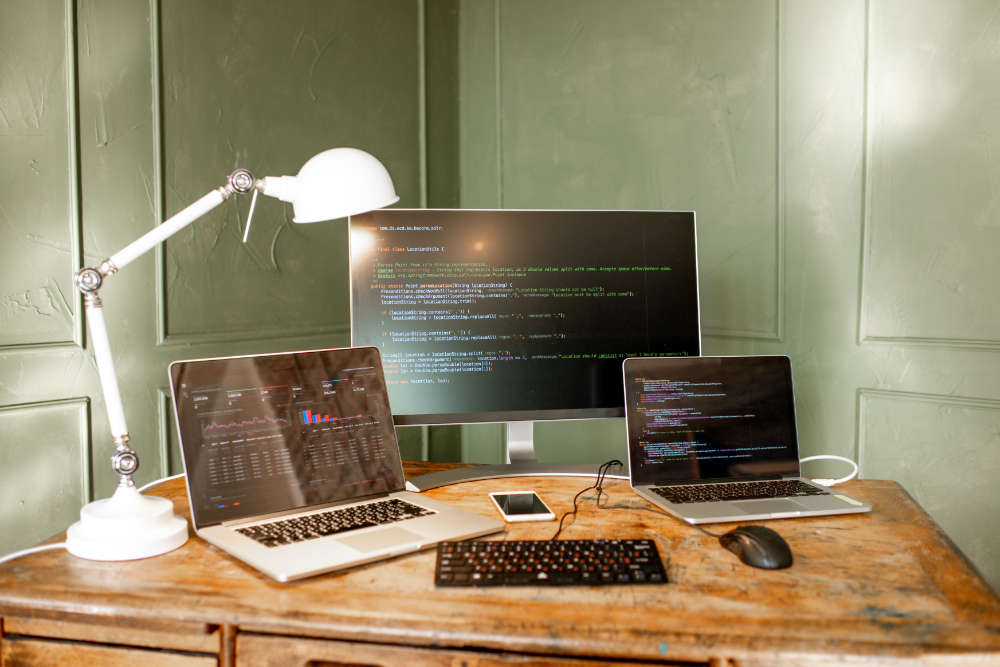
To learn if linear programming can be negative, you first need to know the basics.
However, if you just want a straightforward answer, click here, and it will guide you to the exact spot in the article where the answer is.
There are several basic elements of linear programming, and we will explain each of the elements in the following few paragraphs.
Decision variables
The variables in a linear program are a collection of quantities that must be determined to solve the issue.
You can solve the problem after establishing the variables’ optimal values.
Because it must be decided what value each variable should have, the variables are referred to as decision variables.
The variables often state how much of a resource to use or how much of an activity to engage in.
For instance, a variable can indicate how many acres should be cleared from a particular forest area during a specific time frame.
Determining the variables in a problem is one of the most complex tasks in linear programming, so don’t get discouraged if you don’t understand it right away.
Objective function
All linear programming issues seek to maximize or minimize a certain numerical number that represents, for example;
- revenue,
- expenditure,
- output volume, etc.
It assesses each choice factor’s contribution to the project or activity’s net present value.
Objective function coefficient
The associated objective function coefficient indicates how much the objective function value would vary if a decision variable’s value were changed by one unit.
Constraints
Take a closer look at the constraints. This one is important for today’s topic!
Constraints are restrictions or limits on the total quantity of a particular resource needed to complete the tasks that determine the degree of success in the decision variables.
All constraints in a linear programming issue are expressed as equations in their standard form.
Non-negative constraints
Whether the goal is to maximize or reduce the net present value of an activity, every decision variable in a linear programming model needs to be positive. This limitation is quite important.
So, there is the answer to the question – no, linear programming cannot be negative. That is because of the non-negative constraints. The variables must meet the non-negativity requirement, which states that they cannot be negative.
Resource Availability & Technological Coefficients
The constraints and non-negativity requirements must be met by a good solution to the linear programming issue.
When the objective function value is the highest, you can find an optimum solution to an LPP with a maximization issue (maximum).
Similar to this, when the objective function value is the lowest, an LPP solution with a minimization issue has an optimum solution (minimum).
An Example of the Elements
To understand the previous explanations, here is a quick example. Follow the model with the knowledge of the paragraph above.
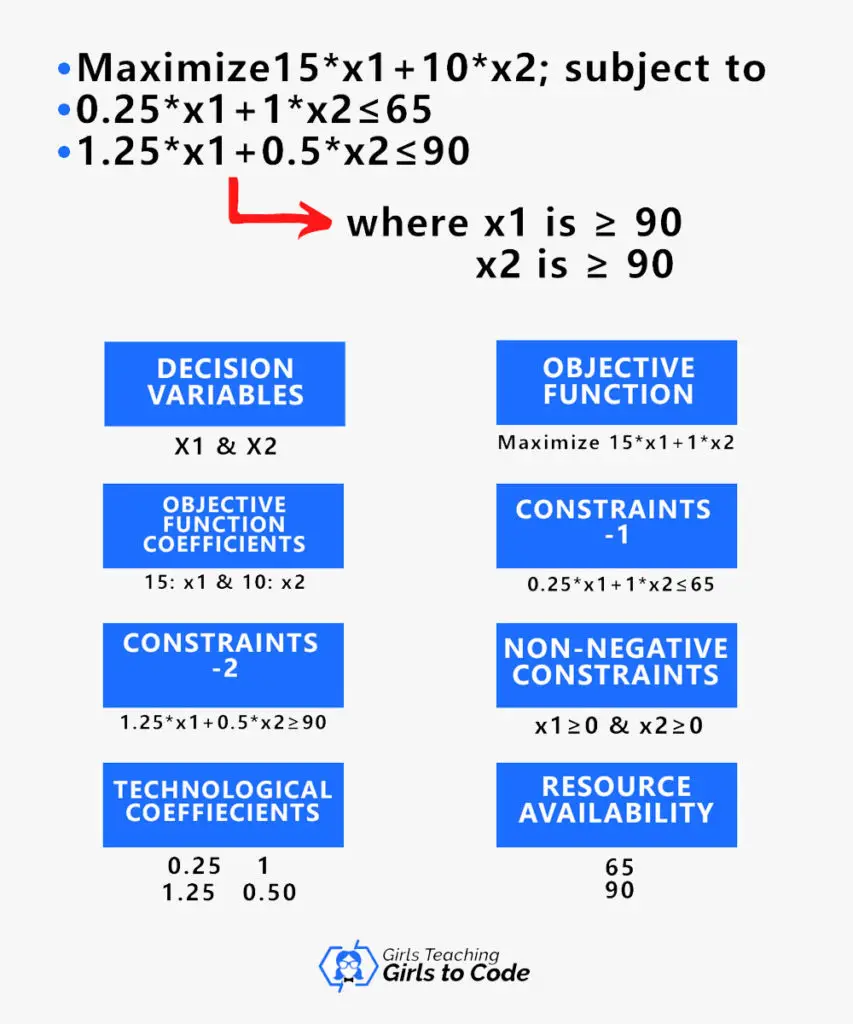
Can Linear Programming Variables Be Negative?
A variable could be negative. You can change the dilemma or problem into one with just non-negative variables if at least one of the variables is negative (0 to inclusive).
As a result, you still retain the traditional form.
Let’s follow the example.
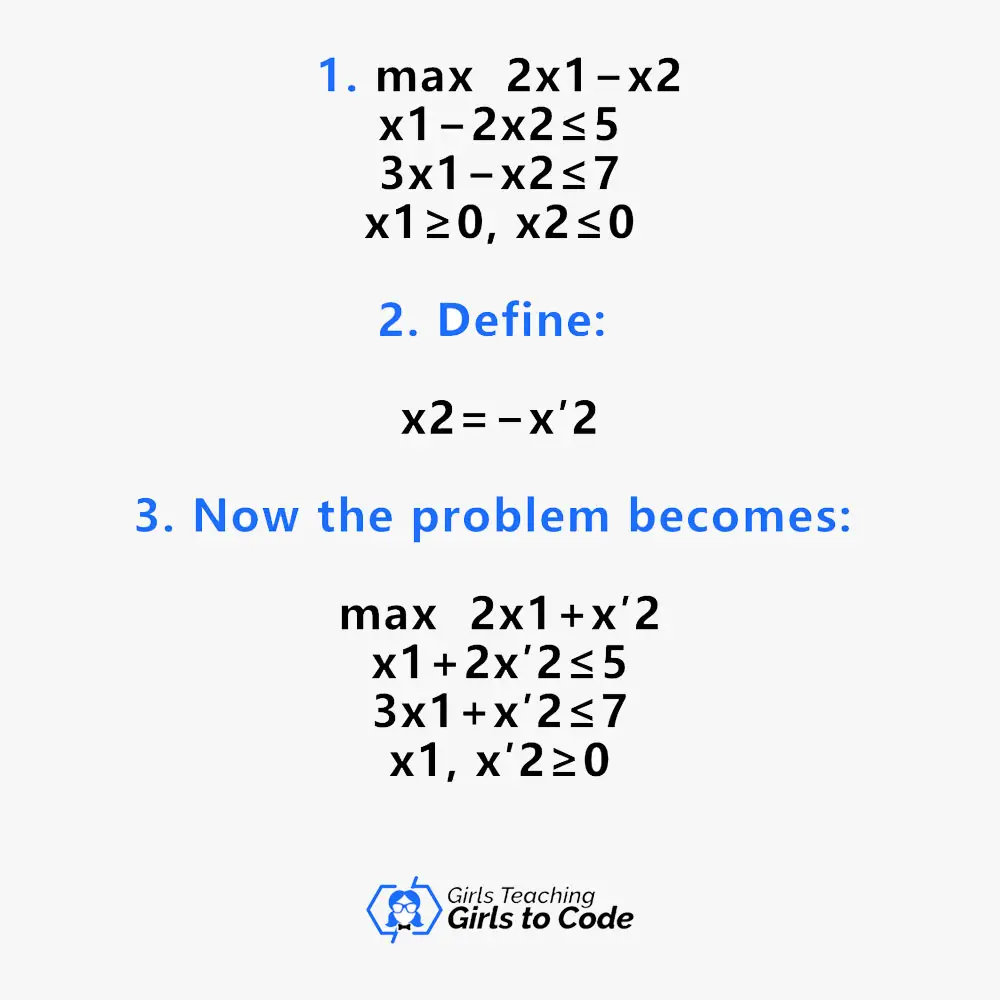
Can An Optimal Solution Be Negative?
First, let’s find out what the optimal solution is.
A linear program’s optimum solution meets all constraints with either the largest or least value of the objective function.
To put it another way, we are given an objective function and certain restrictions, and we must determine the lowest or maximum values in a linear programming problem.
Following applying the constraints, we are left with a viable area. That area is the collection of all solutions that satisfy all of the criteria.
The solution that yields the largest or smallest objective function value using the region’s extreme points is now the optimal solution.
Every linear program has an extreme point that is the best solution, but finding it is not always possible, so have that in mind!
An optimum is if a solution’s values are larger than or equal to zero. The best solution is one where all values are larger than or equal to one. If there are negative numbers, the solution is still not ideal, and you must choose a new pivot point in the matrix.
But, the fact is that every linear program has an extreme point that is an optimal solution.
What Are Some Limitations of Linear Programming?
There are seven limitations of linear programming (LP).
1. It isn’t easy to express an objective function in mathematical form.
2. Even after determining the objective function, it might be challenging to identify:
- social,
- institutional,
- economical, and other limitations.
3. It’s also conceivable that linear equality equations may not precisely specify the objective function and limitations.
4. One of the primary issues with LP is figuring out the pertinent values of the co-efficient of restrictions.
5. LP’s presumptions are similarly irrational. It presupposes that the factory percentage stays the same. It also assumes a linear association between the:
- variables,
- production and cost,
- production and
- overall income.
All of these presumptions presuppose perfect market competition and consistent returns to scale.
However, in reality, the relationships are not always linear, and the market is characterized by imperfect competition.
6. Because it uses mathematical principles, it is a reasonably sophisticated procedure.
It is challenging to identify optimal solutions to various business challenges using LP models since they provide a trial-and-error approach.
7. In linear programming, the amount of all inputs must be increased in a set proportion to increase production by a single process.
However, by only raising one or two inputs, it is possible to boost the output of various items somewhat.
It implies that production may be somewhat boosted by changing the proportion of multiple elements.
Conclusion
There is a lot to learn when it comes to linear programming! But, hopefully, now you understand it a lot better!
If you still didn’t, check other posts on Linear Programming to enrich your linear programming knowledge!
Advertising links are marked with *. We receive a small commission on sales, nothing changes for you.